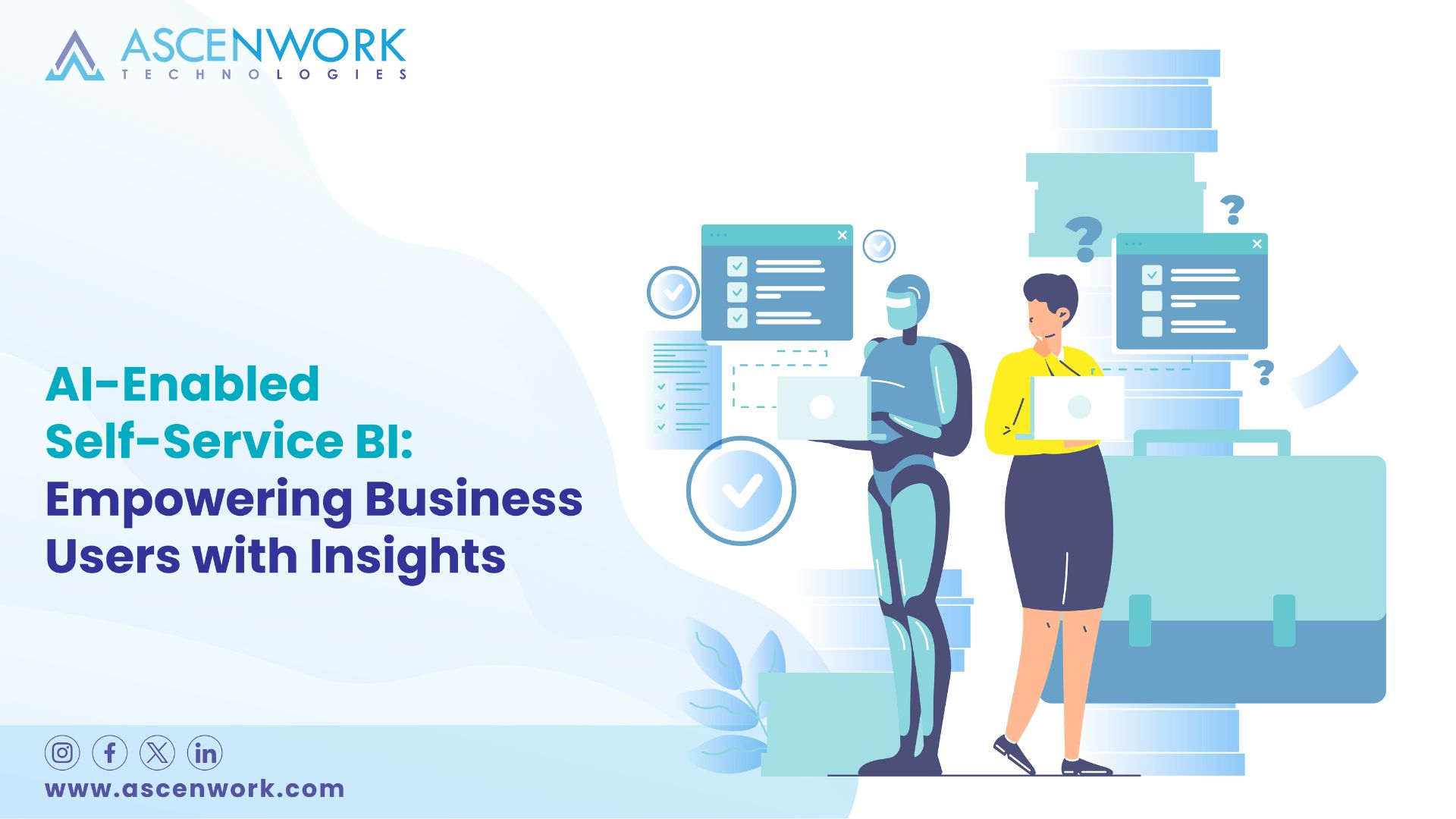
In today’s data-driven landscape, AI-Enabled Self-Service Business Intelligence (BI) has emerged as a transformative force, empowering business users with actionable insights. Leveraging artificial intelligence, this innovative approach enables users to access, analyze, and visualize data autonomously, fostering informed decision-making across organizations. Discover how AI-driven BI revolutionizes data accessibility and amplifies business agility in this comprehensive exploration.
Key elements of AI-driven Business Intelligence include
Key elements of AI-driven Business Intelligence include:
- Data Integration and Preparation: AI-driven BI relies on integrating data from various sources such as databases, cloud services, IoT devices, and more. This data needs to be cleansed, transformed, and prepared for analysis.
- Machine Learning Algorithms: AI-driven BI employs a variety of machine learning algorithms to analyze data and discover patterns, trends, and insights that may not be immediately apparent to human analysts. These algorithms include regression, classification, clustering, and deep learning models.
- Natural Language Processing (NLP): NLP enables AI-driven BI systems to understand and interpret human language. This capability allows users to interact with the BI system using natural language queries and receive insights in a format that is easy to understand.
- Predictive Analytics: AI-driven BI systems leverage predictive analytics to forecast future trends and outcomes based on historical data and current conditions. Predictive models help businesses anticipate changes in customer behavior, market dynamics, and other key factors that impact business performance.
- Advanced Visualization: Visualization plays a crucial role in AI-driven BI by presenting complex data analysis results in intuitive and interactive visual formats. Advanced visualization techniques such as heatmaps, scatter plots, and network graphs help users explore data relationships and gain deeper insights.
- Real-time Analytics: AI-driven BI enables real-time analysis of streaming data from various sources such as social media, sensors, and transactional systems. Real-time analytics empower businesses to make timely decisions and respond quickly to changing conditions in their environment.
- Automation and Decision Support: AI-driven BI systems automate routine tasks such as data collection, analysis, and reporting, freeing up human analysts to focus on more strategic activities. Moreover, these systems provide decision support by recommending actions based on data insights and business objectives.
- Data Security and Privacy: AI-driven BI solutions must prioritize data security and privacy to ensure that sensitive information is protected against unauthorized access, breaches, and misuse. This involves implementing robust encryption, access controls, and compliance with data protection regulations such as GDPR and CCPA.
- Scalability and Flexibility: As data volumes and user demands grow, AI-driven BI systems need to scale seamlessly to accommodate increased workloads and evolving business requirements. Scalability and flexibility are essential for ensuring that the BI solution remains effective and efficient over time.
- Continuous Improvement and Optimization: AI-driven BI is an iterative process that requires ongoing monitoring, evaluation, and refinement. Businesses must continuously assess the performance of their BI systems, identify areas for improvement, and incorporate feedback to enhance accuracy, relevance, and usability.
By integrating these key elements, organizations can harness the power of AI-driven Business Intelligence to gain valuable insights, drive informed decision-making, and gain a competitive edge in today’s data-driven economy.
Examples of AI-driven BI tools
Examples of AI-driven Business Intelligence (BI) tools abound in the modern landscape, reflecting the integration of artificial intelligence into analytics platforms to enhance decision-making processes and glean deeper insights from data. Here are several notable examples:
- Tableau: Tableau, a widely-used BI tool, has incorporated AI capabilities through its natural language processing (NLP) feature, Ask Data. This functionality enables users to pose questions in plain language, with AI algorithms parsing through data to generate relevant visualizations and insights.
- Power BI: Microsoft’s Power BI leverages AI to streamline data preparation, analysis, and visualization tasks. Its AI-driven features include natural language query (Q&A), which allows users to interact with data using everyday language, and AI visuals that automatically select the most appropriate chart types based on the data.
- Qlik Sense: Qlik Sense employs AI and machine learning algorithms to facilitate data exploration and discovery. Its Cognitive Engine assists users in uncovering hidden patterns, associations, and outliers within datasets, enabling more informed decision-making.
- ThoughtSpot: ThoughtSpot offers an AI-driven analytics platform that enables users to search for insights using natural language queries. Its SpotIQ technology automates the process of analyzing data, generating insights, and presenting relevant visualizations, empowering users to make data-driven decisions rapidly.
- Sisense: Sisense utilizes AI and machine learning to enhance data analysis and provide actionable insights. Its augmented analytics capabilities enable users to identify trends, correlations, and anomalies within their data, facilitating proactive decision-making across various business functions.
- Domo: Domo’s BI platform integrates AI and machine learning to automate data preparation, analysis, and reporting tasks. Its AI-driven insights engine surfaces relevant KPIs, trends, and anomalies in real-time, empowering users to derive actionable insights from their data quickly.
- Yellowfin BI: Yellowfin BI incorporates AI-driven features such as automated data discovery, anomaly detection, and natural language processing to accelerate the insights-to-action process. Its AI capabilities enable users to uncover valuable insights from complex datasets and drive business outcomes effectively.
Key aspects and functionalities of AI-driven BI tools
Key aspects and functionalities of AI-driven Business Intelligence (BI) tools encompass a range of capabilities designed to enhance data analysis, decision-making, and insights generation. Here are several key aspects and functionalities:
- Data Integration and Preparation: AI-driven BI tools streamline the process of integrating and preparing data from disparate sources. They automate tasks such as data cleansing, normalization, and enrichment, ensuring that data is standardized and ready for analysis.
- Advanced Analytics and Machine Learning: AI-driven BI tools leverage advanced analytics techniques and machine learning algorithms to uncover hidden patterns, correlations, and trends within datasets. These tools can perform predictive analytics, anomaly detection, and clustering analysis to extract valuable insights from complex data.
- Natural Language Processing (NLP): NLP capabilities enable users to interact with BI tools using everyday language. Users can pose questions, make inquiries, and request insights using natural language queries, reducing the barrier to entry for non-technical users and enabling self-service analytics.
- Automated Insights Generation: AI-driven BI tools automate the process of generating insights from data. They can identify trends, outliers, and anomalies within datasets, providing users with actionable insights in real-time. Automated insights generation accelerates the decision-making process and enables users to respond promptly to changing business conditions.
- Cognitive Computing and Augmented Analytics: Cognitive computing capabilities enable BI tools to simulate human thought processes, reasoning, and decision-making. Augmented analytics features assist users in exploring data, identifying patterns, and generating hypotheses, augmenting human intelligence with machine intelligence to drive better decision-making outcomes.
- Personalization and Contextualization: AI-driven BI tools offer personalized experiences tailored to the specific needs and preferences of individual users. They can deliver customized dashboards, reports, and visualizations based on user roles, interests, and objectives. Contextualization features ensure that insights are presented in the relevant business context, enabling users to derive actionable insights more effectively.
- Predictive and Prescriptive Analytics: AI-driven BI tools enable organizations to move beyond descriptive analytics to predictive and prescriptive analytics. They can forecast future trends, anticipate customer behavior, and recommend optimal courses of action based on historical data and predictive models. Predictive and prescriptive analytics empower organizations to proactively address challenges and capitalize on emerging opportunities.
- Data Visualization and Storytelling: Data visualization features enable users to create interactive charts, graphs, and visualizations that communicate insights effectively. AI-driven BI tools facilitate data storytelling by automatically selecting the most appropriate visualizations, highlighting key insights, and guiding users through the narrative arc of the data.
- Real-time Monitoring and Alerts: AI-driven BI tools offer real-time monitoring capabilities that enable organizations to track key performance indicators (KPIs), monitor business processes, and detect deviations from expected norms. They can generate alerts and notifications when predefined thresholds are exceeded or when anomalies are detected, enabling timely intervention and corrective action.
Pros of self-service analytics
Self-service analytics offers numerous benefits to organizations and users alike, empowering them to derive insights and make data-driven decisions more efficiently. Here are some key pros of self-service analytics:
- Empowerment of Business Users: Self-service analytics puts the power of data analysis directly into the hands of business users, reducing their reliance on IT or data specialists. With intuitive interfaces and user-friendly tools, business users can explore data, create visualizations, and extract insights without extensive technical expertise.
- Faster Decision-Making: By enabling business users to access and analyze data on their own, self-service analytics accelerates the decision-making process. Users can quickly generate reports, dashboards, and ad-hoc analyses to respond to evolving business needs and market dynamics in real-time.
- Increased Agility: Self-service analytics promotes organizational agility by allowing users to adapt to changing requirements and explore new datasets without waiting for IT or data teams to intervene. This agility enables businesses to stay responsive to market trends, competitive pressures, and customer preferences.
- Enhanced Data Exploration: Self-service analytics tools provide users with the flexibility to explore data from multiple angles and perspectives, fostering a deeper understanding of underlying trends, patterns, and correlations. By empowering users to ask and answer their own questions, organizations can uncover valuable insights that may have otherwise remained hidden.
- Reduced Bottlenecks: Traditional analytics processes often involve lengthy queues and bottlenecks as business users submit data requests to IT or data teams. Self-service analytics eliminates these bottlenecks by enabling users to access and analyze data independently, freeing up IT resources to focus on more strategic initiatives.
- Improved Collaboration: Self-service analytics encourages collaboration and knowledge sharing across departments and teams. By democratizing access to data and insights, organizations can foster a data-driven culture where decision-making is informed by a shared understanding of key metrics, performance indicators, and trends.
- Cost Efficiency: By reducing the reliance on specialized IT and data personnel for routine data analysis tasks, self-service analytics can lead to cost savings for organizations. With user-friendly tools and intuitive interfaces, businesses can maximize the value of their existing data investments while minimizing the need for additional resources.
- Customization and Personalization: Self-service analytics platforms enable users to customize dashboards, reports, and visualizations according to their specific needs and preferences. This level of customization empowers users to tailor insights to their unique requirements, driving more informed and personalized decision-making.
FAQ
- What is AI-driven Business Intelligence
: – AI-driven Business Intelligence (BI) refers to the integration of artificial intelligence (AI) technologies into BI systems and processes. It involves using AI algorithms and techniques to automate data analysis, generate insights, and facilitate decision-making processes within organizations. AI-driven BI systems leverage machine learning, natural language processing, and other AI capabilities to enhance data processing, identify patterns, predict trends, and deliver actionable insights from large and complex datasets.
- What is self-service analytics?
: – Self-service analytics is an approach to data analysis that empowers users to access, analyze, and visualize data without relying heavily on IT or data professionals. It allows business users to explore data, create reports, and generate insights independently using intuitive and user-friendly analytics tools. Self-service analytics platforms typically feature drag-and-drop interfaces, pre-built templates, and interactive visualization capabilities that enable users to derive insights from data with minimal technical expertise.
- What exactly is Self-Service Business Intelligence
: – Self-Service Business Intelligence (BI) refers to the ability of business users to independently access and analyze data using BI tools and platforms without extensive support from IT or data specialists. It enables users to explore data, create customized reports, and derive actionable insights to support decision-making processes. Self-service BI platforms typically offer intuitive interfaces, interactive visualizations, and ad-hoc querying capabilities that empower users to explore data and gain valuable insights without relying on technical assistance.
- How is AI used in BI?
: – AI is used in Business Intelligence (BI) to enhance data analysis, automate repetitive tasks, and uncover valuable insights from large and complex datasets. AI-driven BI systems leverage machine learning algorithms, natural language processing, and predictive analytics techniques to automate data preparation, identify patterns, detect anomalies, and generate actionable insights. AI in BI enables organizations to streamline decision-making processes, improve operational efficiency, and gain competitive advantages by leveraging the power of data-driven insights.
- How is AI used in databases?
: – AI is used in databases to optimize performance, enhance security, and enable advanced analytics capabilities. In database management systems (DBMS), AI techniques such as machine learning and natural language processing are employed to automate routine maintenance tasks, optimize query performance, and identify potential security threats. AI-driven database platforms also enable advanced analytics functionalities, such as predictive modeling, anomaly detection, and personalized recommendations, by leveraging AI algorithms to analyze large volumes of data and extract valuable insights for decision-making purposes.