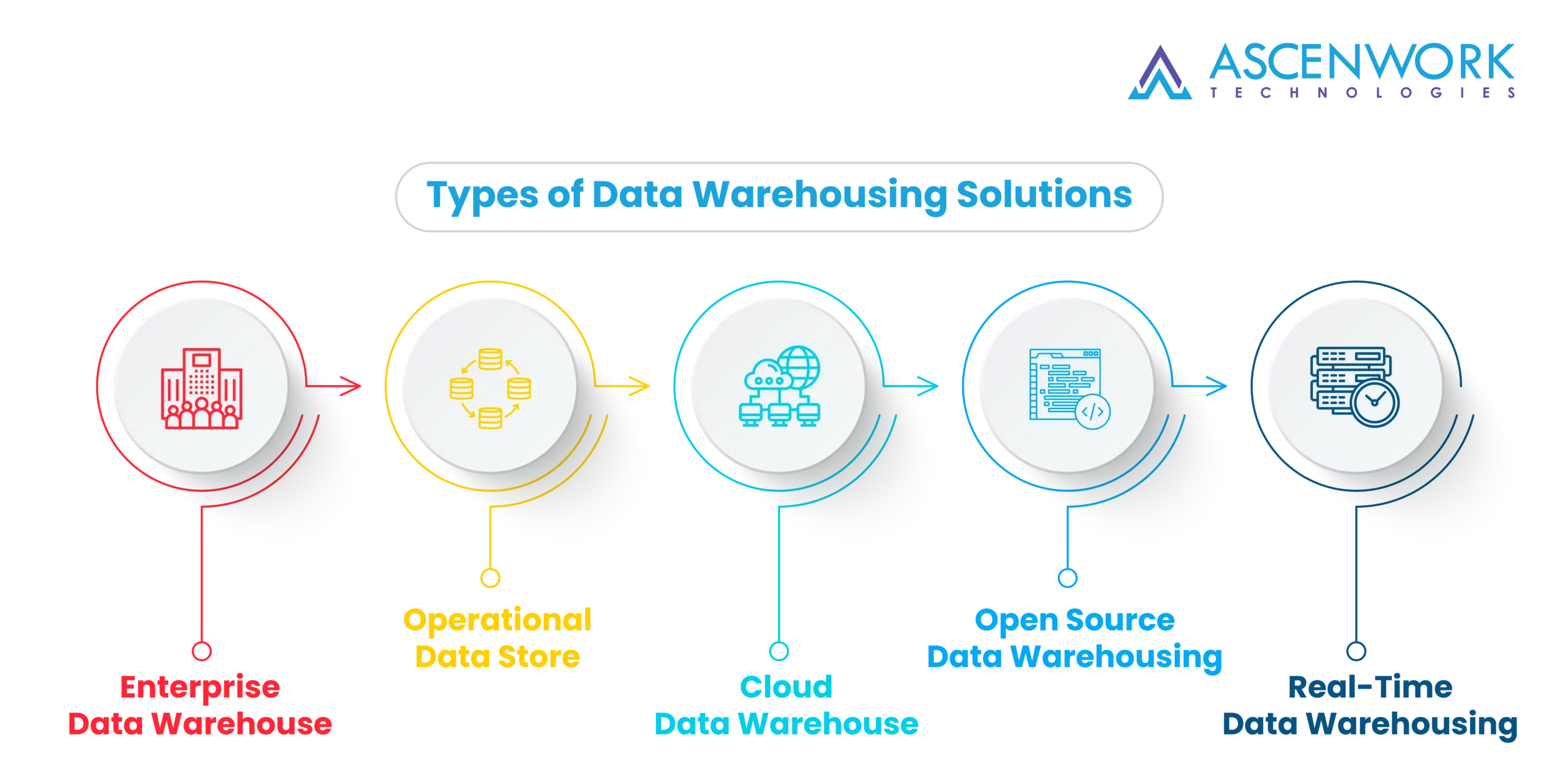
Have you ever pondered the transformative impact that the integration of Machine Learning (ML) and Data Warehousing is exerting on the Information and Technologies industry? Well, let’s dive into the intricacies of this amalgamation, thoroughly examining the significance, advantages, and optimal methodologies that unequivocally render this fusion a revolutionary paradigm shift.
Why Machine Learning in Data Warehousing?
The mutually beneficial interaction between Data Warehousing and Machine Learning (ML) is essential in the field of data management. Sleekly moving away from more conventional methods, ML’s incorporation into Data Warehousing is a major game-changer. Predictive analytics is made easier by this dynamic partnership, which improves decision-making. Also, it increases data processing effectiveness by turning unprocessed data into insights that can be used. In the end, ML and data warehousing work together to simplify processes and enable businesses to fully utilize their data resources, which is a revolutionary development in information management.
Importance of Machine Learning in Data Warehousing
The centrality of ML within the domain of Data Warehousing cannot be overstated. Inextricably linked, ML injects unparalleled value into data repositories. Transitioning beyond conventional norms, its incorporation holds profound significance. ML propels advanced analytics, facilitating the extraction of meaningful patterns from vast datasets. Transitioning to a predictive model, it optimizes decision-making processes. This metamorphosis is pivotal, elevating the importance of ML in Data Warehousing, accentuating the efficiency and strategic prowess of information management systems.
Benefits of Machine Learning in Data Warehousing
Embarking on the integration of ML into Data Warehousing ushers in a cascade of advantages. Seamlessly augmenting traditional systems, ML unfolds a myriad of benefits. Firstly, it enhances predictive analytics, enabling proactive decision-making. Transitioning further, ML optimizes data processing, extracting actionable insights with unparalleled efficiency. This synergy not only fortifies analytical capabilities but also propels strategic initiatives. In essence, the incorporation of ML in Data Warehousing culminates in a spectrum of advantages, shaping a dynamic landscape for data-driven excellence.
Dynamic Adaptation
Within the realm of ML integration into Data Warehousing, dynamic adaptation emerges as a linchpin. Transitioning seamlessly, it fosters a responsive ecosystem. ML’s innate capability to evolve and adjust dynamically ensures optimal performance. This adaptability is quintessential, enabling the system to continually refine its models in response to evolving data landscapes. In essence, the concept of dynamic adaptation in Machine Learning within Data Warehousing propels an environment of perpetual refinement, ensuring sustained relevance and efficacy in the face of ever-changing data dynamics.
Precision and Accuracy
AI Analytics operates as a complex orchestra where data takes center stage. It begins by collecting vast datasets from various sources, ensuring comprehensive coverage. This data is then processed through machine learning algorithms that can identify patterns, relationships, and anomalies. As the future of ai system learns and adapts, it refines its analysis, providing increasingly accurate insights. The beauty of AI Analytics lies in its ability to continuously learn and improve, making it a powerful tool for businesses seeking data-driven decision-making. In essence, it’s a self-taught maestro, orchestrating a symphony of data for optimal results.
Machine Learning in a data warehouse involves integrating ML algorithms to analyze and extract insights from stored data, enhancing predictive analytics and optimizing decision-making processes for improved efficiency.
Machine Learning in data is used to uncover patterns, make predictions, and gain insights. It automates analysis, enhancing decision-making processes and optimizing outcomes in various domains.
AI in data warehousing automates tasks, improves data processing, and enhances analytics. It optimizes storage, aids in decision-making, and enables dynamic adaptation for efficient information management within the warehouse.
Machine Learning involves algorithms that learn patterns from data. An example is spam filters, which learn to differentiate between spam and legitimate emails based on user behavior and content